Cities across the United States are capitalizing on big data. Predictive policing is becoming a prominent tool for public safety in many cities. In Boston, an algorithm helps determine “problem properties” where the city can target interventions. In Chicago, they are protecting citizens by predicting which landlords are not complying with city ordinances. In New York, the Fire Department sends inspectors to the highest risk buildings so they can prevent deadly fires from breaking out.
DrivenData is launching our first civic innovation competition, "Keeping it Fresh," to help cities capitalize on their data.
According to the Centers for Disease Control, more than 48 million Americans per year become sick from food, and an estimated 75% of the outbreaks came from food prepared by caterers, delis, and restaurants. In most cities, health inspections are generally random, which can increase time spent on spot checks at clean restaurants that have been following the rules closely — and missed opportunities to improve health and hygiene at places with more pressing food safety issues.
The goal for this competition is to use data from social media to narrow the search for health code violations in Boston. Competitors will have access to historical hygiene violation records from the City of Boston — a leader in open government data — and Yelp's consumer reviews. The challenge: Figure out the words, phrases, ratings, and patterns that predict violations, and help public health inspectors do their job better.
Winning algorithms will be awarded $5,000 in prizes: the first-place winner will receive $3,000, and two runners-up will receive $1,000 each. But the real prize is the opportunity to help the City of Boston, which is excited to explore ways to integrate the winning algorithm into its day-to-day inspection operations.
Early work on this problem has already indicated that consumers and citizens are leaving clues in their online restaurant reviews. A model from a Yelp internal hackathon tried to predict health scores in San Francisco restaurants. With a simple bag-of-words model, they were able to pick out trends in hygeine scores over time.
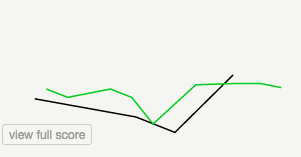
Predictions from Yelp model: Green is predicted, black is inspection results.
A reserach project using Yelp data for the City of Seattle had success picking out patterns that mattered as well. Using a linear classifier, researchers were able to identify severe offenders with 82% accuracy. Their model relied on features built from unigrams, bigrams, and Yelp star-ratings to achieve this result.
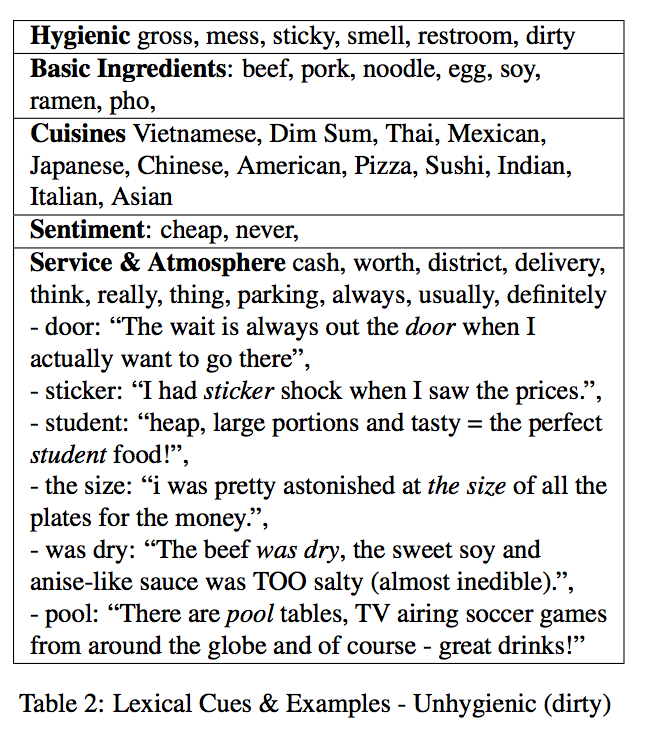
To make things a little more interesting, we got things started on this new set of data for the City of Boston. Using bigram features--that is, pairs of words that appear together--we determined which were correlated with more violations. Bigrams that indicated more violations included:
|
|
And, bigrams that indicated fewer violations included:
|
|
There may be some clues in there, but we're betting you can do better. The competition will accept submissions for eight weeks. Submissions will be evaluated on fresh hygiene inspection results during the six weeks following the competition; after that, the prizes will be awarded. Your submission will not only put you in the running for the prize – it has the chance to transform how city governments ensure public health.
What are you waiting for? These reviews aren't going to parse themselves!